Product Recommendation Engines: What Is It & How It Work
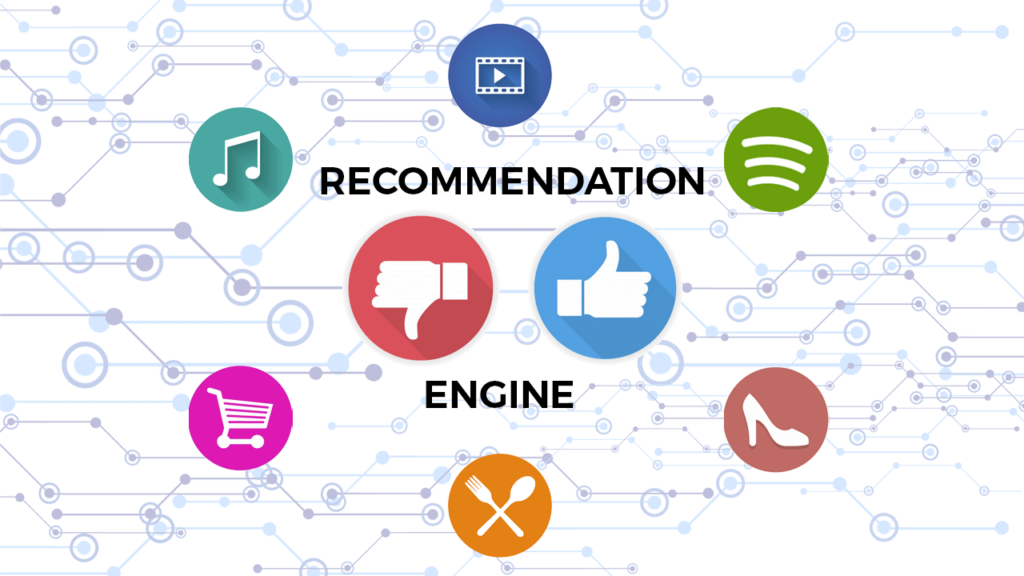
What are Product Recommendation Engines
Product recommendation engines provide users with personalized, contextually relevant recommendations based on their past search behavior or other interactions with your product. This, in turn, leads to greater customer satisfaction and higher conversions for the business.
The benefits that come from using product recommendation engines include increased engagement, increased conversions, more effective targeting; and lower cost per acquisition (CPA).
Types of Product Recommendation Engines
There are three main types of product recommendation engines: collaborative filtering, content-based filtering, and hybrid models.
Collaborative filtering relies on the feedback of other users to recommend products, while content-based filtering looks at the attributes of products to make recommendations. Hybrid models use a combination of both methods.
The best product recommendation engine for your business will depend on your specific needs. Personalized product recommendation engines take into account the individual preferences of each user to make recommendations, while the best product recommendation engines focus on finding the products that are most popular or best rated.
Collaborative Filtering
Sometimes called the “best product recommendation engine,” it is a system that makes suggestions for products, services, or content to users of digital platforms such as e-commerce websites and social networks. The recommendations are based on the preferences of similar users, which the system predicts by using collaborative filtering. It is a method of making recommendations that relies on the collective intelligence of a community of users. The algorithm looks at the items recommended to similar users and then recommends items to the new user that other similar users have also liked.
Content-Based Filtering
Also known as a content-based filtering system, it uses the information it knows about an individual user to make recommendations. The system looks at the user’s past behavior—what products they have purchased, what items they have added to their cart, and what items they have favorited—to understand their interests. It then looks at similar users to find products that those users have purchased, added to their cart, or favorited. Finally, it makes recommendations to the original user based on those findings.
Hybrid Model
A hybrid model combines the collaborative filtering approach with the content-based approach. This system recommends items to users based on the similarity between items as well as the similarity between users. The hybrid model has the ability to recommend items to users even if they have not rated any items yet. This system is more accurate than either of the individual methods alone and can provide better recommendations to users.
How Does a Product Recommendation Engine Work
A product recommendation engine, also known as a recommender system, is an algorithm that makes suggestions for products, services, or content. It relies on feedback data and typically runs using artificial intelligence (AI). AI algorithms analyze user interactions with the site to learn what they like in order to make suggestions.
Data Collection
Product recommendation engines use data to provide personalized recommendations to shoppers. The data used can come from a variety of sources, including a shopper’s past purchase history, items viewed on a website, and even social media activity. This data is then analyzed to identify patterns and trends that can be used to recommend similar or complementary products to the shopper.
Product recommendation engines are a type of artificial intelligence that is used to predict what products a customer is likely to buy. This is done by collecting data about the customer’s past behavior, such as what they have searched for and what they have bought. The data is then analyzed to find patterns that can be used to predict future behavior.
Data Storage
A product recommendation engine is a system that analyzes a customer’s behavior on a website and makes suggestions for other products they might be interested in. This data is stored in a database, which the engine then uses to make recommendations.
There are two types of data that can be used to make recommendations:
1. Purchase data, which tells the engine what products a customer has bought in the past; and
2. Browsing data, which tells the engine what products a customer has been looking at.
Browsing data is generally more effective than purchase data because it can show not only what products a customer is interested in, but also what products they might be interested in but haven’t bought yet.
Data Analysis
Three different types of analysis methods can be used for product recommendations: batch analysis, real-time analysis, and near-real-time system analysis.
1. Batch analysis is the simplest and most common method. It runs on a schedule, typically once a day, and looks at all the data that has been collected since the last time it ran.
2. Real-time analysis is more complex. It looks at data as it comes in and makes recommendations based on that data. This means it can make recommendations based on changes since the last time it ran.
3. Near-real-time system analysis is the most complex. It analyzes streaming data—or any other type of data being received by the system—to provide recommendations as soon as they become available.
Data Filtering
Product recommendation engines use data filtering to take in large amounts of data about products, users, and user behavior. This data is then analyzed to find patterns and relationships. These relationships are used to create recommendations for individual users. The better the recommendations, the more likely a user is to buy a product. One way that this type of engine can recommend new products is by predicting what the customer might like based on their previous purchases.
For example, if a customer bought a car that was similar to another car they were considering, it would be reasonable to assume that they would also like that car too.
How Product Recommendation Engines Ensure Seamless Shopping Experience
A product recommendation engine (PRE) is a system that recommends items to users of digital platforms such as e-commerce websites and mobile apps. By understanding the user’s needs and preferences, a product recommendation engine can provide personalized recommendations that help the user find the right product faster and more easily. A well-designed product recommendation engine can increase conversions, repeat purchases, and customer satisfaction.
In an era where customers want instant gratification with the tap of a finger or swipe of their phone screen, a product recommendation engine can be an invaluable asset to any business—not just in retail but also in the services and entertainment industries too. The convenience factor makes it one of the most effective ways for merchants to increase sales, and generate higher profits. The best ones seamlessly personalize every interaction by analyzing data from customer’s browsing patterns and transactions so they know what offers will resonate most with them.
As consumers shop online at increasingly high rates, these products offer merchants increased opportunities for conversion. They allow brands to offer cross-selling through hyperlinks embedded in emails, providing greater insight into a consumer’s purchase history when suggesting additional products they might like while shopping on other sites, giving businesses the opportunity to retarget ads across channels with automated notifications when similar products are purchased elsewhere, driving traffic to particular items based on social media engagement and geographical data about what products certain shoppers seem drawn towards; and so much more!
Frequently Asked Questions
Where are recommendation engines used?
Product recommendation engines are commonly used on e-commerce websites and in apps to provide personalized recommendations to users. They can also be used on content websites to recommend similar or recommended articles.
Which algorithm is used in the product recommendation system?
The algorithm used in a product recommendation system looks at the past behavior of users to predict what they might want in the future. It relies on user feedback to improve its recommendations over time.
How do recommendations increase sales?
Product recommendation engines, also known as recommender systems, are a subset of artificial intelligence used to make suggestions for products, services, potential friends, or content.